Benefits of Tracking Equipment Downtime
You have machines on your plant floor. Sometimes they are up and sometimes they are down. Hopefully, when you expect them to be up, they are up and performing the way they are supposed to; meaning performing as expected, producing an acceptable amount of scrap, etc. But, what if they’re not and you’re facing unplanned machine downtime?
This a major part of manufacturing. Knowing when the machines are running and when they’re not – and if they are running – are they performing the way they are designed to perform? Unfortunately, this equation hasn’t traditionally been easy for manufacturers to solve.
What’s more, many manufacturers see reports on paper that they know aren’t reflective of truth on the plant floor. This means that it’s being reported that machines are up when they’re not or performing better than they actually are. This is because of things like shortstops or downtime that fails to be reported, due to manual processes or improper recordings.
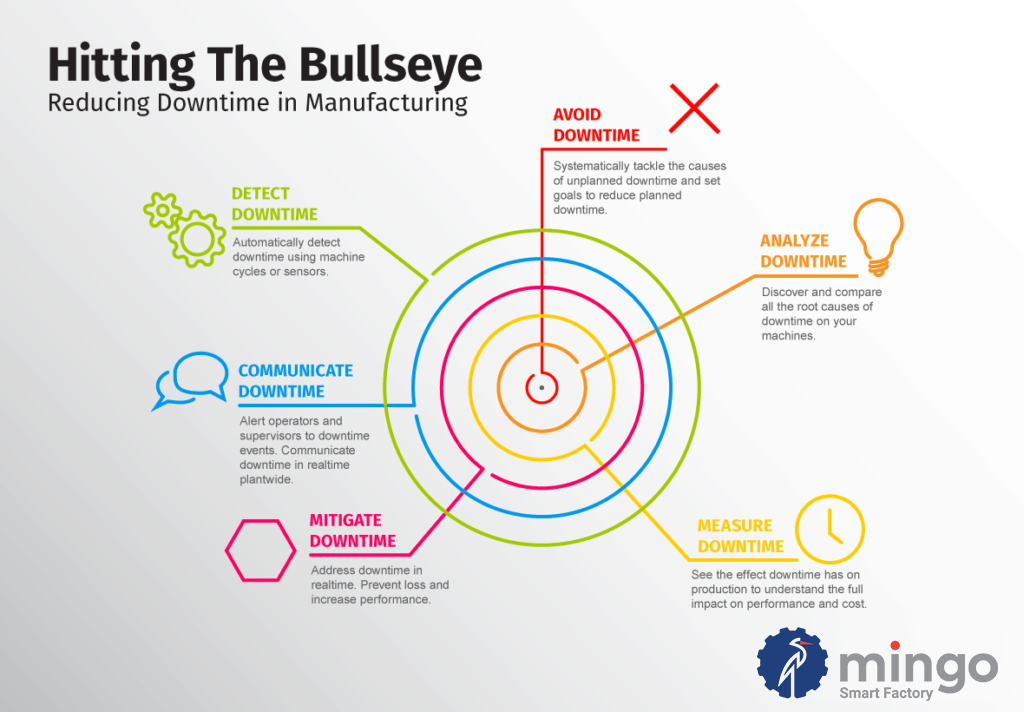
“How Do I Conduct a Machine Downtime Analysis?”
If you’re experiencing a loss of productivity because of downtime, you are looking for answers. “How much downtime am I experiencing? What is causing downtime and why? How much is it costing me?” An accurate analysis of your downtime will increase capacity and throughput because your machines will be running more often.
A downtime analysis isn’t easy if you aren’t tracking the right things, but this blog will outline the basics for an effective downtime analysis and will answer the question of “How to log and track machine downtime?” and “How to analyze unplanned and planned machine downtime?”.
How To Track Downtime Correctly?
If you are tracking it at all, congratulations. But just to revisit this, you should be tracking downtime in this way:
- Machine/Cell/Line
- What machine is down?
- Whether it was unplanned or planned
- Was the downtime scheduled?
- Downtime by minute
- How many minutes was the machine down?
- Shift and operator
- Who was running the machine?
- Category
- What is the specific cause of the downtime?
There are two other methods in tracking downtime which are useful in the long term, but when just getting started, are not necessary:
- Department
- Who brought the machine down? Maintenance? Production Support?
- Cost of machine downtime
- Did the downtime cause other machines to go down or stop production? If so, what were the number of units that went unproduced, and what profit did it cost?
“How Do We Ensure that the Downtime Data We Have is Accurate?”
There’s a saying in marksmanship that when shooting a rifle “precision leads to accuracy”. The basic premise is that a rifleman who consistently puts a group of shots on target, and close together, even without hitting a bullseye is better than a shooter whose shots are all over the place but occasionally hits a bullseye.
The first example demonstrates technique and skill and with a slight mechanical adjustment will consistently and accurately hit their mark, while the other has either undisciplined talent or dumb luck- you’ll never know what you are going to get.
The same rings true in manufacturing analytics. For years, plant managers used the traditional dumb luck methods of downtime tracking in which operators self-reported every hour on the hour their production numbers and downtime. It has proved to be a hit-or-miss affair and one that doesn’t help in consistently reducing downtime.
Downtime Reporting with Machines and Operators
Just self-reporting downtime, it turns out, represents a triple threat to plant floor visibility:
• It is inconsistent (many downtime events go unrecorded)
• It is inaccurate (many downtime intervals are mistimed)
• It is ineffective (many or all downtime reasons are missing)
There are a lot of reasons why an operator might misreport on downtime and we’ll spare the reader our deep philosophical insights into the human mind or musings on human frailty. Needless to say, it is an entirely predictable human problem and is in fact, fixable.
The question shouldn’t be, “How do I accurately track machine downtime?”, but rather “How do I accurately report on downtime?”. By counting machine cycles we can see when a machine is down, but it won’t tell us why it is down. To do that you need consistent, reliable operator input.
How To Log Machine Downtime?
Differentiate between planned and unplanned to log machine production downtime. Roundup and track downtime by minutes to simplify your data collection. Many manufacturers choose an arbitrary threshold of a few minutes so they only focus on significant events. This is understandable when looking at downtime hour by hour, but over the course of a shift or a full week, these “micro stops” or “shortstops” can add up.
Case Study: H&T, the largest battery component manufacturer in the world experienced this first hand. Microstoppages were causing up to 18 hours of unplanned downtime every week. After implementing Mingo in the plant, H&T reduced microstoppages by 71% and overall downtime by 73%.
With these things identified, you should be able to start analyzing your daily downtime by focusing only on the downtime events that impacted productivity and cost significantly.
“How Do I Start Analyzing Machine Downtime?”
Start with a 24-hour period from your latest downtime logs or downtime report. This will focus your analysis on a manageable time period and provide you with enough data to gain insights and prepare for the next day’s starting shift. Start with unplanned downtime, since while planned time can still be reduced, it is the unplanned downtime that can create cascading issues to performance and impact productivity unexpectedly.
Look hour by hour to understand when and how long downtime occurred. This will provide you with an understanding of what might have caused or exacerbated downtime. Was it caused by the confusion of a shift change or an unscheduled operator break?
“What Is Causing My Machines To Go Down?”
Look at the machines which were down during the shift. Was the downtime unplanned or planned? Was it brought down by maintenance or operations? Catalog all instances of downtime so you can compare categories. (We really don’t condone doing this in Excel, but for some, it may give you a good baseline to use what you learn and apply it to a machine downtime tracking Excel document for making improvements.)
Why Use a Pareto Chart to Analyze Downtime?
Drill down to your top 5 or so biggest chunks of downtime. A helpful tool is a Pareto chart, named because Vilfredo Pareto identified the 80/20 rule and created the chart that bears his name. By plotting your downtime in a Pareto chart you can determine the 20% of issues that cause 80% of downtime. These are the critical areas you need to address.
Our example Pareto chart shows downtime in minutes and it is easy to see that 80% of our machine downtime is caused by 3 reasons:
- End of Shift Cleaning – 75 minutes
- Short Stop – 46 minutes
- Dial-In – 40 minutes
With End of Shift Cleaning taking up most of our time reducing End of Shift Cleaning will have the greatest time impact on our production. But is this the right thing to focus on?
In this table we can see 3 things:
- Number of downtime events by reason
- Total duration in minutes
- Average duration in minutes
We have a lot of shortstops and almost the same amount of time in Dial-In. Our production teams may be able to completely eliminate both of these problems and give us back 86 minutes of production time.
How to Mitigate Downtime by Department?
Sub-divide your downtime if it is initiated by the operator, production support, or maintenance. This will allow you to pinpoint who is responsible for the downtime and avoid the blame game. If unplanned downtime was caused by an operator error or a series of unplanned micro stops, then use it to flag that behavior and better understand what is happening.
For unplanned maintenance, could the downtime have been avoided if the operator was better trained? Flag your unplanned maintenance time if it was justified or not. This will lead to better qualifications of downtime later on.
The Goal: Reduce or Eliminate Machine Downtime
The goal here is to consistently, accurately, and effectively track downtime, so we can stop it when it happens, see its impact on performance, understand what causes it, and avoid it in the future. (And, you can use this blog as a machine downtime tracking template.) That means categorizing every meaningful downtime event faithfully and correctly.
Remember our target? We don’t want to just see machine downtime when it happens, we want to understand downtime so we can stop it from happening now and in the future. It starts with reliable detection of downtime, but it doesn’t stop there. Without identifying its root causes, we still won’t understand how to prevent it.
Automate Downtime Detection and Ensure Operator Reporting
1. Automatically Detect All Downtime
To avoid misreporting on downtime, start tracking all downtime automatically by measuring machine cycles. You’ll have an accurate gauge of the number of downtime events and their length.
2. Communicate Downtime Plant-Wide
Provide triggered mobile alerts and real-time dashboards and scoreboards to visibility to the entire plant. Knowing is half the battle.
3. Mitigate Downtime
With the clock ticking, the operator is encouraged to fix the downtime issue immediately.
4. Measure All Downtime
Prompt the operator to select a downtime category so the event has a reason and its impact can be understood fully, especially if it’s a recurring problem.
5. Analyze Downtime
Determine which downtime reasons are preventable and take action to prevent them in the future.
6. Avoid Future Downtime
Systematically tackle the issues causing unplanned downtime. Set goals and implement processes to reduce planned downtime.
Machine Data Insights on Downtime Only Matter When This Happens
So, what have really good manufacturers done to get better data and increase uptime and improve performance?
Step 1: They Understand Their Plant Floor
One of the number one things I hear over and over again from manufacturers that have solved these problems (truly solved them) is that they fixed the “everybody is wrong” problem. What I mean by this is that often all the different departments have different data and are acting on it (or not acting on it).
For example, the plant floor may know that the 3rd shift always starts late. Additionally, the staff on that shift hasn’t been trained as well as some of the others, so they have more shortstops (breaks, etc.) than the other shifts. They may choose to under-report this to align with other shift numbers. Unfortunately, when things are manually recorded these shortstops are left off the final reports and it looks as though the 3rd shift performs exactly like the others in the data, but they don’t.
Later, when reports are pulled, some of the information will show that downtime and production took a big hit during the 3rd shift for several months. When they go to see why they won’t find a reason in the data, but in reality, we know an issue exists.
Furthermore, even if we did see the issue in the data, we would be finding out months too late to do anything about it.
The manufacturers that do this best understand that all the data needs to line up. That means all the machine data needs to fit two pieces of very essential criteria.
The machine data must be accurate and, the machine data must be central to every department.
Step 2: Everyone Sees the Same Thing
That second point at the end of step 1 is really what a lot of top manufacturers have in common. Even though automating all data collection from machines offers massive help in this, there are manufacturers that rely on manual data collection (even if it’s not always timely or accurate) that are still able to achieve this and see the same benefits as the manufacturers that automate machine data collection.
Centralizing all of the data – making a single source of information available to every department – can solve a lot of the problems from our earlier example. If we knew that downtime and under-production were issues on the 3rd shift, and everyone had access to this data, we could see that trend developing very quickly in our dashboards; as opposed to months down the line after the business had already lost money.
We could work backward to identify the source of the trends. Providing a single system to put data into can also help streamline manual data entry processes to ensure everyone is entering data the same way. This kind of consistency can help improve accuracy as well.
Step 3: More Automation and More Data
Lastly, what I see manufacturers who are really excelling at this doing is automating processes and data collection. Those two things go hand and hand; as I have written about before. The more you automate the more data you need to make sure you don’t experience major issues.
Having data automated from machines and centralized in a single place for every department can provide unprecedented success and ROI inside of the organization. Manufacturers can see issues in real-time, understand trends quickly, diagnose the root of downtime in a second, and improve the OEE of underperforming machine cells.
This is because centralized and automated data is more accurate and more available. If it is presented in a consumable form to staff, there are few things that offer the ability to impact a manufacturing business more, at least from a cost perspective, than really good machine data insights.
Accurate Data Contributes to Success
Most manufacturers have these problems to some degree. Understanding machine downtime really comes down to have good accurate data from machines, and then getting that data into the hands of the people that can solve issues that stick out. These are the types of organizational cultures that find success with manufacturing analytics. Neither of these things occurs when data isn’t centralized or consistently collected.
Manufacturing analytics applications like, Mingo, offer major ROI for manufacturers that struggle with these kinds of issues.